Applications of Machine learning in Digital marketing 2024
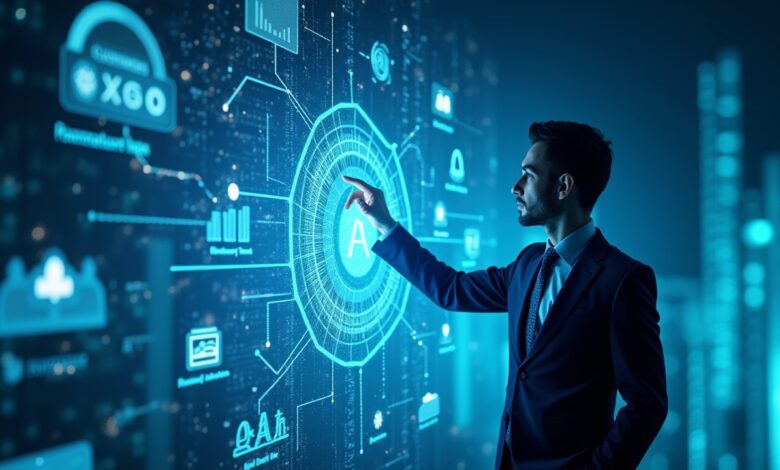
Machine learning is transforming the way you approach digital marketing. This technology analyzes vast amounts of data to help you understand customer behaviors and preferences. With powerful algorithms, machine learning predicts trends and tailors marketing strategies, allowing you to enhance your campaigns effectively.
You may already be familiar with how companies like Netflix and Amazon use machine learning to personalize user experiences. These insights can guide your marketing efforts, whether you are experienced or just starting. By using machine learning, you can improve engagement and increase sales by making well-informed decisions based on data.
Key Takeaways
- Machine learning helps predict customer behavior through data analysis.
- Personalized marketing strategies can enhance customer engagement.
- Effective use of machine learning can lead to increased sales.
The Role of Machine Learning in Digital Marketing
Personalized Marketing Applications
Machine learning helps tailor marketing efforts to individual preferences. For example, streaming platforms like Netflix use algorithms to suggest shows based on your viewing history. E-commerce sites like Amazon recommend products by analyzing what you have browsed or purchased before. These tools make your experience more engaging and relevant.
Predictive Analytics Usage
Predictive analytics uses past data to forecast future trends. By analyzing patterns from customer interactions, you can better predict what your audience wants. This helps you create targeted campaigns that are more likely to lead to sales. Companies that excel in using this tool can enhance their marketing strategies effectively.
Data-Driven Marketing Decisions
With machine learning, you can make informed decisions based on data instead of guesswork. By gathering insights from various sources, you can group customers with similar interests and behaviors. This kind of segmentation allows you to craft specific messages that resonate with each group, improving your chances of conversion significantly.
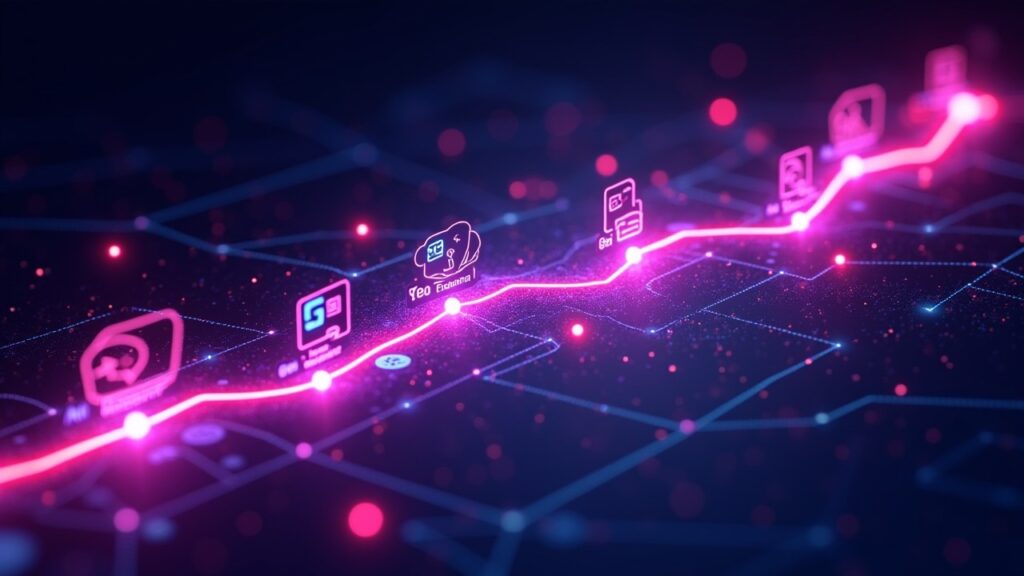
Machine Learning Concepts in Marketing
Supervised vs Unsupervised Learning
In marketing, you often encounter two main types of machine learning: supervised and unsupervised learning. Supervised learning uses labeled data to train algorithms. This means that the data is already sorted into categories. For example, you might use labeled customer data to identify who is likely to make a purchase.
Unsupervised learning, on the other hand, works with unlabeled data. It looks for patterns and similarities without prior categories. This method can help identify groups of customers based on behaviors or interests, allowing for better-targeted marketing strategies.
Neural Networks and Engagement Prediction
Neural networks mimic the human brain’s structure and function. These systems are commonly used to predict customer engagement by analyzing various data points. For example, they can track how users interact with content, which can help you understand what keeps customers interested and engaged.
By leveraging neural networks, you can improve your marketing campaigns by tailoring content that resonates with your audience, ultimately enhancing customer interactions.
Natural Language Processing for Customer Insights
Natural language processing (NLP) focuses on how to analyze and understand human language. This technology can be incredibly useful in marketing. You can use NLP to evaluate customer feedback and reviews to gain insights into their feelings and preferences.
By understanding customer sentiment through their words, you can create more relevant and effective marketing messages that resonate with your target audience.
Reinforcement Learning for Content Recommendation
Reinforcement learning teaches machines to make decisions by learning from trial and error. This approach is especially useful in recommending content or products based on previous customer interactions. As your customers engage with your offerings, the system learns what they like and adjusts recommendations accordingly.
Utilizing reinforcement learning can lead to a more personalized experience for your audience, making it easier for them to find what they want while improving their overall satisfaction with your marketing efforts.
Advanced Applications of Machine Learning in Digital Marketing
Machine learning goes beyond basic data analysis, offering marketers a toolkit of advanced methods for refining campaigns, improving ad targeting, and predicting customer behavior. Here are ways to take full advantage of machine learning in digital marketing, with a focus on how it can improve your strategies for 2024.
Deep Learning Marketing for Enhanced Customer Insights
Deep learning, a subset of machine learning, dives deeper into complex patterns and massive data sets to extract insights that traditional algorithms may miss. For example, by analyzing subtle behavior patterns, deep learning marketing allows you to predict which users are likely to purchase soon, enabling you to serve them the right content at the right time. This predictive power is invaluable when designing campaigns that resonate on a personal level, enhancing user engagement and conversion rates.
Deep learning also allows you to make real-time adjustments. For instance, if a user frequently abandons their shopping cart, you can use deep learning algorithms to identify triggers for this behavior, then tailor your retargeting ads to address these concerns directly. This application of machine learning in digital marketing takes engagement and conversions to new levels by continuously refining messaging based on individual customer behavior.
Machine Learning in Advertising: Real-Time Ad Optimization
Machine learning in advertising goes beyond simply placing ads. It enables real-time optimization of ads based on metrics like click-through rates, engagement levels, and conversion likelihood. Platforms like Google Ads and Facebook Ads already incorporate machine learning to optimize ad placements, but with your own tailored machine learning strategies, you can gain even more control.
For example, machine learning algorithms can detect the best-performing ad formats, placements, and timing for your unique audience. This means that instead of relying solely on platform-level data, you’re able to make more refined decisions about ad spend and campaign design. Additionally, machine learning advertising methods can analyze competitors’ strategies, identifying gaps where your ads might perform better. This insight is key to staying competitive, particularly in saturated markets.
Integrating Artificial Intelligence and Machine Learning in Digital Marketing
When combined, artificial intelligence (AI) and machine learning open the door to predictive marketing, customer service automation, and content customization. AI-driven chatbots, for example, are becoming essential in customer support and lead generation. These bots analyze past interactions and learn to respond in more human-like ways over time, enhancing customer satisfaction.
In digital marketing, AI can assist with sentiment analysis. By monitoring social media and customer feedback, AI identifies emerging trends and customer opinions in real-time, so you can adjust your campaigns accordingly. AI and machine learning together create a powerful system that not only responds to trends but also anticipates future needs. For instance, if you’re running a campaign during a holiday season, AI can predict which products will sell best, enabling you to allocate resources more effectively.
How to Use Machine Learning in Digital Marketing
- Predictive Customer Segmentation
Moving beyond traditional segmentation, machine learning segments customers based on likely future behaviors rather than just past interactions. This allows you to prioritize high-value customers, target at-risk users, and adjust your strategies accordingly. - Dynamic Content Creation
Use machine learning to analyze what type of content each segment responds to best. With this data, you can dynamically adjust your website content, emails, and ads. For example, machine learning can recommend specific blog topics that will engage your audience based on their search behaviors, helping you maintain relevance and drive traffic. - Advanced Retargeting
By examining user behavior patterns, machine learning improves retargeting ads to reach users at the right point in their journey. For instance, if a user viewed a product but didn’t purchase, machine learning identifies the optimal time and platform for re-engagement. - Voice Search Optimization
Voice search is growing, and machine learning helps optimize content for this format by analyzing user speech patterns and predicting search trends. Voice search relies on natural language, so optimizing your content for conversational queries can help you capture this audience.
What Is Machine Learning in Digital Marketing?
Machine learning is the backbone of a data-driven marketing strategy, analyzing large data sets to detect patterns and predict outcomes. By leveraging this technology, you can make informed decisions based on predictive models. This isn’t just about guessing what might happen – it’s about using real data to predict future trends, creating a more responsive and proactive approach to your campaigns.
For example, machine learning algorithms can review thousands of variables, including purchase history, online behavior, and demographic information, to determine which products a specific audience is likely to buy. As a result, your marketing efforts become more targeted and efficient.
How Machine Learning Is Applied in Digital Marketing Today
Machine learning applications are diverse and constantly evolving, but here are some top ways they’re applied in 2024:
- Behavioral Targeting
Machine learning algorithms detect micro-behaviors that indicate purchase intent. For example, if a user frequently clicks on eco-friendly products, you can use this insight to tailor your messaging and recommend similar items. - Sentiment Analysis
By analyzing social media conversations and reviews, machine learning gauges how customers feel about your brand. This real-time feedback allows you to adjust marketing messages or even the product itself, improving customer satisfaction and brand perception. - Real-Time Bidding (RTB)
RTB uses machine learning to optimize ad placements in real time. Based on factors like bid history and user profiles, machine learning helps you secure the best placements at the lowest possible cost. This is crucial for brands looking to maximize their advertising budgets while reaching the most relevant audience.
Digital Marketing in the Artificial Intelligence and Machine Learning Age
In the digital marketing world of 2024, AI and machine learning have transformed how brands connect with customers. Rather than pushing generic messages, you can now deliver experiences that feel tailored to each user. This technology goes beyond content personalization; it encompasses every touchpoint, from how users discover your brand to how they receive support post-purchase.
AI-powered recommendation engines, like those on Spotify or YouTube, demonstrate how machine learning can keep users engaged. By studying past interactions, these engines suggest relevant content, helping brands retain customer attention. Whether you’re in retail, media, or any other sector, using machine learning for digital marketing lets you create a similarly engaging journey for your users.
Getting Started: Your Roadmap to Machine Learning Success in Digital Marketing
- Invest in Quality Data
Machine learning depends on quality data. Ensure your data collection is consistent and accurate, as poor-quality data will lead to flawed predictions. - Identify Specific Goals
Define what you want to achieve with machine learning. Are you trying to improve ad efficiency, increase engagement, or boost sales? Setting clear goals will guide your model selection and help you measure success accurately. - Select the Right Tools
From Google Cloud AI to Amazon Machine Learning, choose tools that align with your goals and expertise level. Many of these platforms offer user-friendly interfaces, enabling you to experiment without needing a full data science team. - Monitor and Adjust
Machine learning models require continuous evaluation. Track performance and adjust as necessary, whether that means updating data inputs or refining your algorithms.
Machine learning is no longer an option but a necessity for digital marketers looking to stay competitive. It offers a profound understanding of your audience, allowing you to deliver personalized, impactful marketing that resonates. By integrating machine learning into your digital marketing strategies in 2024, you’re not only adapting to the future but also creating campaigns that truly connect with your customers.
you may also like: Artificial Intelligence in Education: the Future of Teaching and Learning
One Comment